Call us toll-free: 800-878-7828 — Monday - Friday — 8AM - 5PM EST
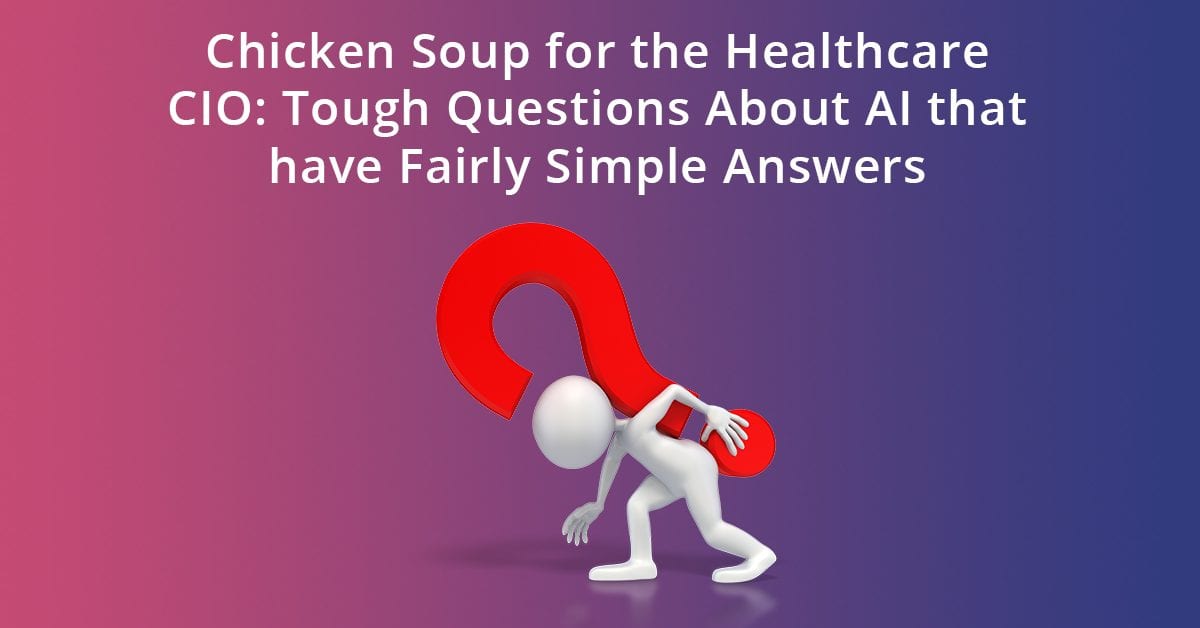
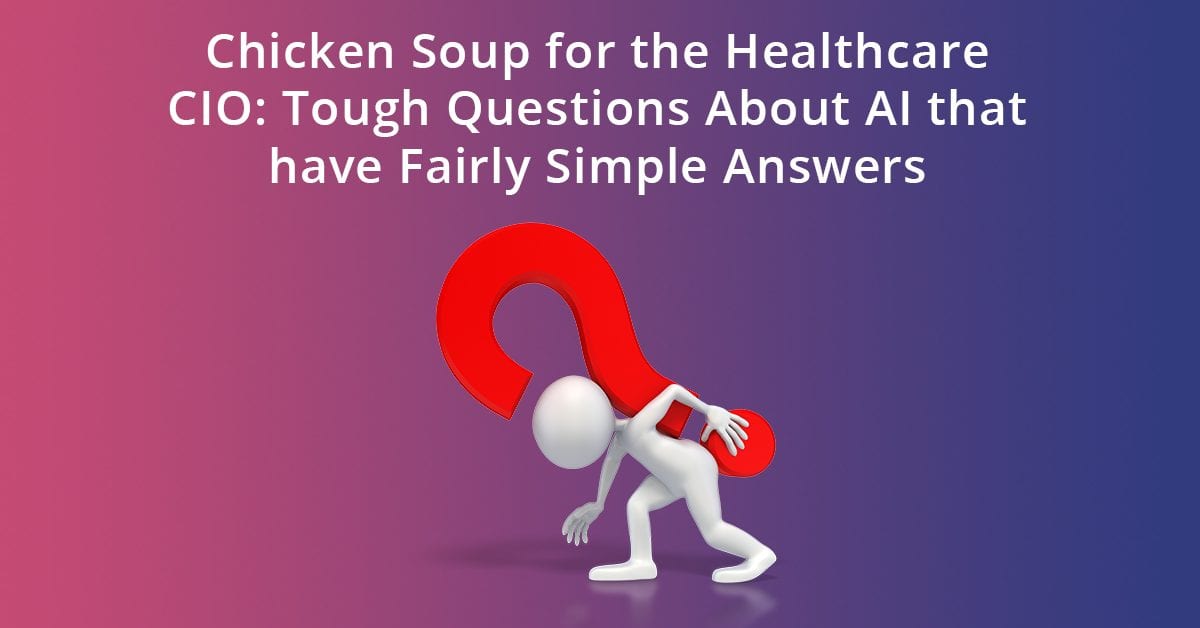
Chicken soup for the healthcare CIO: tough questions about AI that have fairly simple answers
Tumultuous… the word that best describes the hope, expectations and trepidations regarding artificial intelligence (AI) for healthcare CIOs across the globe today. Traditionally in healthcare, CIOs have been viewed by their medically trained counterparts and CEOs as system implementers. Only recently with data centers the size of football fields, full of data that knows all and tells all, are health systems finally changing the CIO’s role to fit within the same Venn diagram as that of the Chief Medical Officer and the Chief Financial Officer. Slowly, the role that was identified as the top most job for an IT consultant climbing the career ladder in the 1990s has begun to evolve, turn strategic, and become accountable to identify new ways of saving the organization from digital doom while the rest of the organization focuses on saving lives.
What has AI got to do with this? Well, traditional digital infrastructure improvement dollars have run out Physicians are overloaded with data entry. Nurses are scrambling to enter orders in an electronic mess of records. Patients are using Google, asking a ton of questions, and playing doctor with the actual doctor and the doctor can’t keep up.
Enter the desire to explore if the historic volumes of data available to the organization could make the health system smarter, more efficient, and perhaps even, dare we say, “futuristic” like AI is doing to publishing and print media, or entertainment or transportation. So now every CIO wants to experiment with AI.
AI has become the new buzzword in the healthcare industry and everyone wants to get started on an AI initiative without a clear idea of how that provides a clear ROI. Making the investment into AI is non-trivial and poses several unanswered questions that hold the health systems back from tapping into the value of their own data and realizing the true potential. Recently, several advanced health systems such as Rush University Medical Center, Duke Health, and UNC Health Care are already claiming AI-driven victory, and have been recognized as stage 7 mature in their analytics by organizations such as HIMSS. But many others are grappling with the fundamentals, owing to a lack of clarity of direction.
Enter the “new” healthcare CIO. With increasing expectations to be a strategic leader within their system, the healthcare CIO is the new superhero. Yet, even superheroes have questions. Here are some common ones we attempt to answer to drive forward the discussion between CIOs and the healthcare AI community.
How much data is too little and too less?
Ideally, a predictive AI platform powered by machine learning (ML) does not need a football field of data. Depending on the use cases, one can begin a trial of the very first AI solution with as little as two to three years of historic health system data that is easy to extract. The machine learning models will typically use a well-established method called cross validation to ensure that models are being trained to learn from data but the performance measures are validated on a blind set. An established norm is to use a subset 70% of this data to train the predictive models and remaining 30% to test the accuracy of the model. Then create several such subsets and validate the performance results by holding out and doing blind tests over the entire dataset. The data can be as simple as what is readily available in the EMR systems, operational schedules, billing, and other health IT systems already in place as part of the digital transformation strategy. Gradually and steadily, over a period of time, health systems have the option of exploring more data assets and appropriate use cases by adding more data sources like billings, claims, pharmacy, psychosocial and even data that is obtained from wearable devices. Start with a use case that is easy to measure and implement and delivers ROI quickly. We recommend looking at use cases such as reducing unwarranted variation, ED census estimation, or ED Short Stay (LWBS) prediction or improving patient flow. For a health system, these data assets are plentiful, and operational rollout can be as simple as adding one field within current systems. They’re quick to adapt, implement and have a visible effect on your operations and patient outcomes resulting in significant savings.
How do I make it simple for my care teams to use and reduce the effort on their end?
The right AI platform integrates back into existing workflows, which means the care teams don’t need to go out of their way to understand and derive the insights that are being provided. Operationalizing AI can be challenging in the beginning, but it does not take intense effort or time from the care teams. On the contrary, with the first visible win, the AI platform becomes an integral part of the health system, an entity that teams depend strongly on to make the right decisions. Moreover, AI is a journey and it begins with a buy-in. It takes multiple teams within the health system to come together and understand that moving forward means cruising the ship towards greater outcomes. Most chief medical officers and physicians today acknowledge that they need to invest time in understanding the use of AI in the system.
How do I trust the predictions from my healthcare AI or ML models?
Much work remains in this field to make healthcare AI truly assistive. It is always hard to trust predictions that are derived from a machine, however, there is a movement afoot to make explainable AI easier to digest and simpler to accept. Explainable AI for Healthcare allows care coordinators and physicians to understand, as well as view how the model arrived at its decision, making ML and AI more efficient and accountable. Regardless, AI needs to be viewed not as Artificial Intelligence but as Assistive Intelligence that augments and supports the human decision-making process. The role of AI is not to make autonomous decisions but helps care teams make better decisions faster and in a more effective manner.
What about AI governance and its impact?
The ethics of AI has been a fairly well debated topic over the last few years. While the impact and implications of AI on lives are being consistently looked at, AI governance and consent over data usage, especially at a national or global scale, is a factor organizations are working on. With GDPR, HIPPA and other data regulatory acts that help keep the use of data in check, there is hope that AI will continue to be used in a manner that is fair and transparent. With additional security measures taken to ensure that the data never leaves the system of the healthcare organization, the right AI platform will ensure that they have safety measures in check until a larger governing body is able construct regulations to include the use of AI. Data governance may start small, but has the potential to be mighty and drive real transformation.
When is the right time to invest in AI for healthcare?
Ideally, yesterday, but it is never too late to start. According to a report by NewVantage Partners, nearly 80% of healthcare execs are investing more in big data and AI, and it doesn’t take much to get started. Find a partner who understands what use case is necessary for you to begin and who is able to look into your data to identify those underlying patterns that help save millions of dollars. With the right partner helping you implement your AI solution, your AI implementation can literally fund itself. So an investment today can yield returns in as little as three months, allowing the CIO to demonstrate an immediate and visible win.
Finding true north when it comes to AI is a formula that healthcare organizations are still trying to decipher, but many of them are close. It’s only a matter of time before someone is able to pen down the “Healthcare AI Manifesto” allowing everyone to follow a set of steady steps that leads to success. But until then, you’re either trying and getting on board the AI train, or become a laggard and left behind.
We prefer the former.