Call us toll-free: 800-878-7828 — Monday - Friday — 8AM - 5PM EST
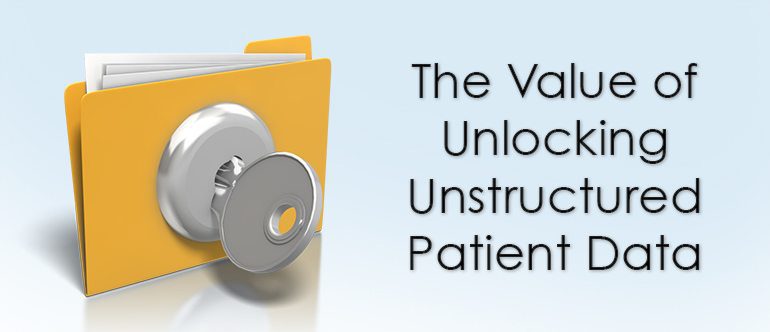
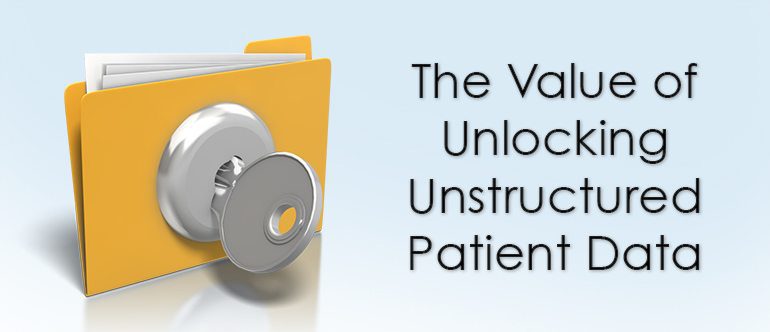
Written by John Smithwick for Healthcare Intelligence Network
Getting patients diagnosed correctly and treated appropriately depends on providers gathering both quantitative data, which is typically structured, and qualitative data, which is typically unstructured. When comparing both types of data, it’s more challenging to manage and derive value from unstructured data.
Structured data is that which is quantifiable and measureable, such as signs like lab results, blood sugar levels and cholesterol. This type of data is objective and can be entered discretely into EMRs via predefined fields. Since the data is structured, software systems are able to understand the meaning of the data, interpret the data and report on it. Structured data can be put to use by clinicians at the point of care to aid their decision-making.
On the other hand qualitative data, such as symptoms like pain, discomfort and fatigue, is considered unstructured data. This type of data is subjective to the patient and is often gathered through conversations based on what the clinician asks and what the patient discloses. While it might seem like a simple exchange of niceties between clinician and patient, these communications provide a lot of information to the clinician, such as whether a patient is experiencing depression, or notices shortness of breath upon standing.
Correct diagnoses and appropriate treatments are dependent on managing both structured and unstructured data. Managing each type of data appropriately also greatly influences the outcomes a healthcare organization is able to deliver. As the amount of reimbursements tied to outcomes increases, delivering quality outcomes becomes all the more important.
Problems with Unstructured Data
Managing structured data is usually handled well. It’s in the management of unstructured data where problems arise. There are two primary problems with unstructured data:
- The first problem is technical. Unstructured data is most often recorded in EMRs in free text fields or note fields. Data stored this way makes it very difficult for software systems to interpret, understand and analyze.
- The second problem is process-related. Unstructured data is not gathered consistently or systematically. A clinician only knows about symptoms if he or she asks the patient, which doesn’t always happen, or if the patient discloses the information, which, again, doesn’t always happen. When symptoms are overlooked or patients withhold information, clinicians can’t make the right diagnosis or give the best treatment.
The ballooning amount of data available is its own issue. In 2012, worldwide digital healthcare data was estimated to be equal to 500 petabytes, and that number is only growing: the data is expected to reach 25,000 petabytes in 2020. It can be a daunting challenge for healthcare organizations to gain value from this mountain of data. To add to this, industry consensus is that approximately 80 percent of all healthcare data is unstructured data.
What the Future Holds for Unstructured Data
So, what if technology could not only ensure patients were being diagnosed correctly, but also automate the process? Cloud-based care management and patient engagement software are providing new ways for healthcare organizations to unlock the value of unstructured data. In essence, by creating “structured symptoms”—gathering patient-reported symptoms and discretely capturing them in a way the data could be analyzed.
These platforms systematically assess patients for symptoms (and signs) that they might not get asked about directly by a provider, and that they might not self-disclose because they don’t think it’s important (or because they simply forget). Care management software then stores patient symptom information in a structured way, allowing this previously unstructured data to be analyzed and made actionable.
The bottom line? Whether gathered via care management software, EHR or patient-specific physician insights, all data should be structured and be ready for interpretation and analysis. This is especially critical in value-based models: for any risk-bearing entity, getting this complete picture is absolutely critical in order to give patients the right treatment at the right time, to improve outcomes and prevent adverse health events.